In an era defined by data-driven decision making, the significance of historical data in the development of quantitative models cannot be overstated. This rich tapestry of past information serves as the backbone of predictive analytics, guiding analysts and researchers to unearth patterns and trends that may otherwise remain obscured.
By diving deep into historical records, we can glean insights into complex systems, be it in finance, healthcare, or environmental science. Each data point tells a story, one that weaves through time and informs our understanding of future possibilities.
Yet, the challenge remains in sifting through the vast ocean of data, where noise often masquerades as meaningful signal. As quantitative modeling continues to evolve, the ability to harness this historical reservoir becomes crucial, not only enhancing predictive accuracy but also empowering decision-makers to navigate uncertainty with confidence.
The Significance of Historical Data
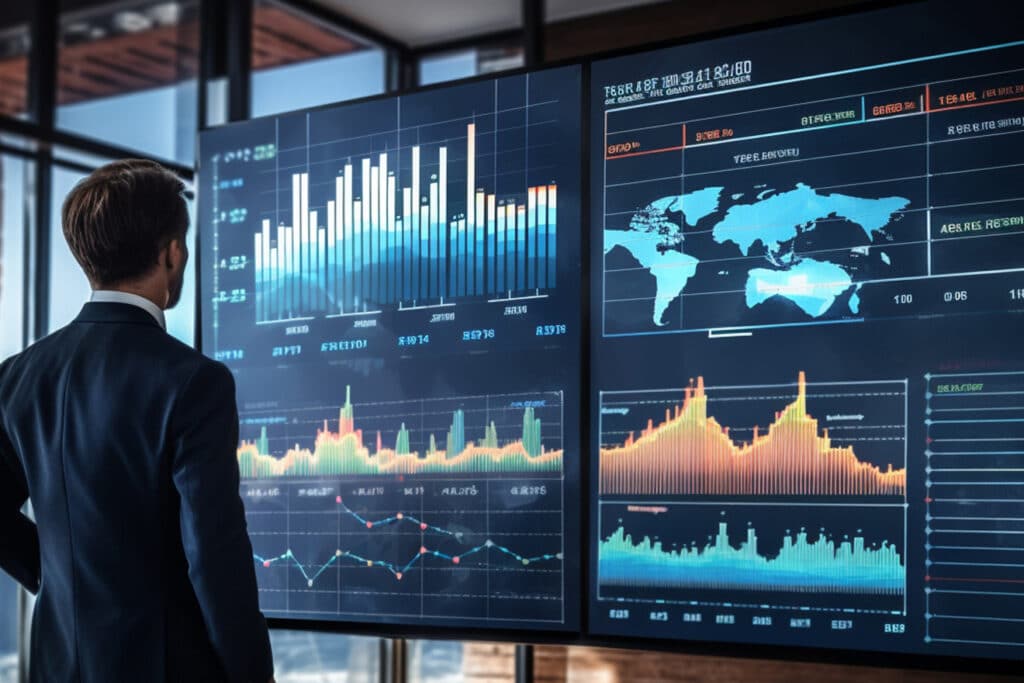
The significance of historical data in developing quantitative models cannot be overstated; it serves as the backbone of informed decision-making in a myriad of fields, from finance to climate science. Historical data offers a rich tapestry of insights, weaving together past trends, anomalies, and behaviors that can illuminate the path forward.
Tools like free replay chart elevate this analysis by allowing users to interactively revisit and analyze historical market data, providing a hands-on approach to understanding past price movements and market dynamics. By scrutinizing years, sometimes decades of data, analysts can uncover patterns that might otherwise go unnoticed—trends that ebb and flow like tides, influencing everything from market forecasts to resource management strategies.
The ability to quantify and analyze these historical nuances not only enhances predictive accuracy but also empowers decision-makers to mitigate risk and seize opportunities with greater confidence. Furthermore, the interplay of diverse datasets often reveals unexpected correlations, making the past not just a record to learn from, but a vital tool to navigate future uncertainties.
Data Preprocessing Techniques
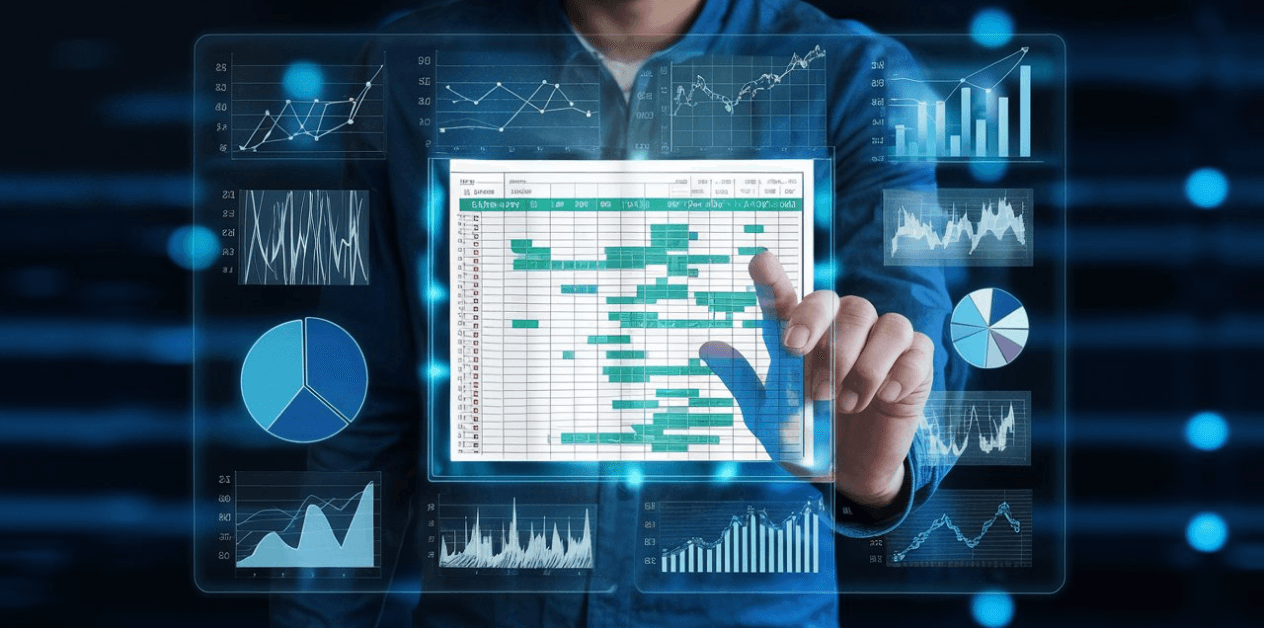
Data preprocessing techniques are vital in transforming raw historical data into a structured format that can be effectively utilized in quantitative models. This multifaceted process encompasses a variety of steps, including data cleaning to remove inconsistencies and errors, normalization to scale the data appropriately, and feature selection to identify the most significant predictors influencing outcomes.
Each of these techniques can seem unassuming, yet they play a pivotal role in enhancing model accuracy. For instance, while imputation techniques fill in missing values, they also prevent the loss of potentially valuable information, underscoring the importance of careful handling.
Moreover, encoding categorical variables through one-hot encoding or label encoding ensures that the model can interpret non-numerical data, bridging the gap between human intuition and machine learning algorithms. Ultimately, the efficacy of any quantitative model hinges on the rigorous application of these data preprocessing strategies, allowing for a more nuanced understanding of historical trends and facilitating robust predictions.
Conclusion
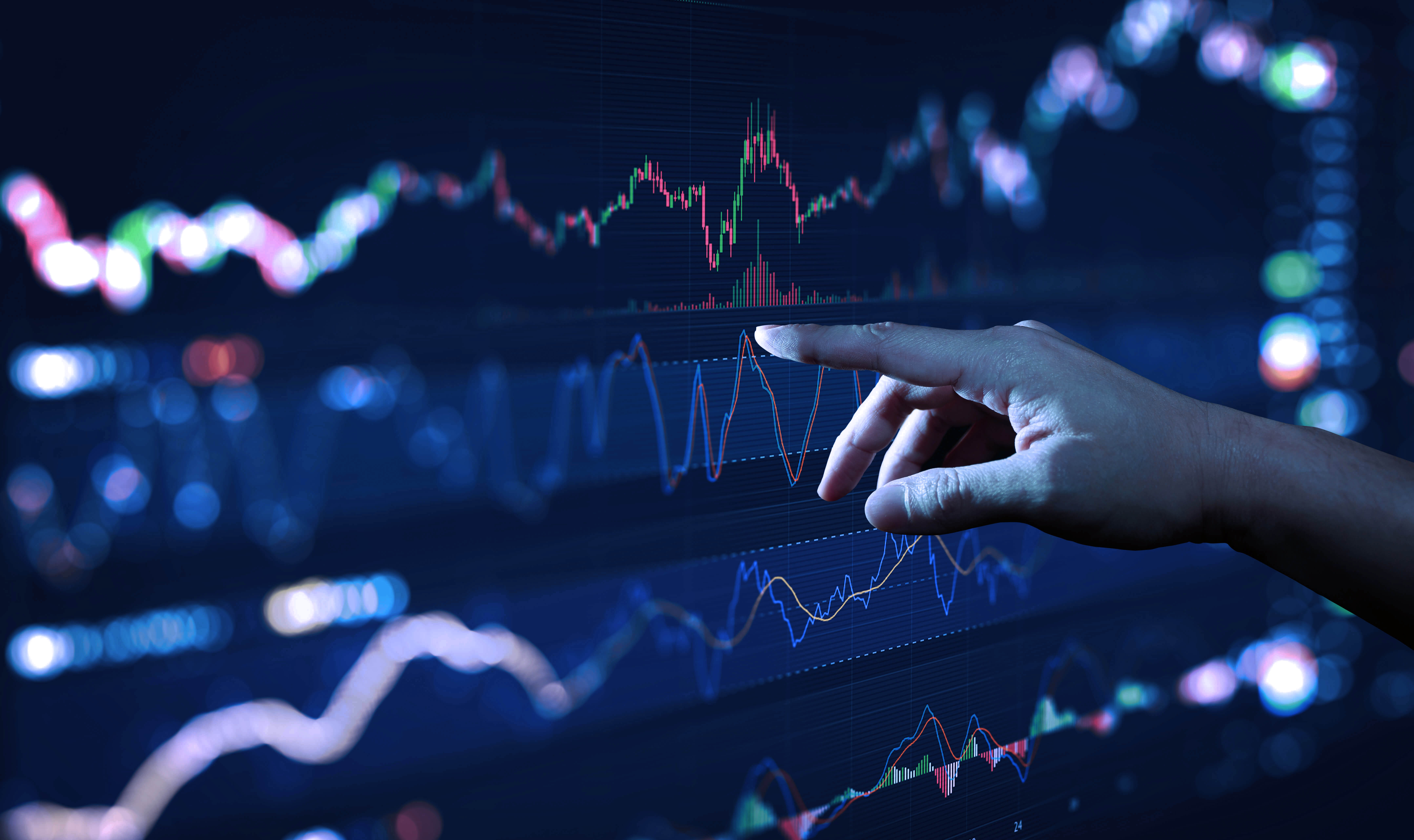
In conclusion, the integration of historical data into the development of quantitative models is indispensable for crafting robust and reliable forecasts across various fields, including finance, economics, and environmental science. By analyzing past patterns and trends, practitioners can enhance their understanding of complex systems and improve decision-making processes. The use of tools such as a free replay chart can further enrich this analysis, allowing users to visualize changes over time and refine their models accordingly.
Ultimately, leveraging historical data not only provides a solid foundation for creating quantitative models but also ensures that these models are well-equipped to adapt to future uncertainties and dynamics.